Overcoming the data scarcity dilemma in machine learning
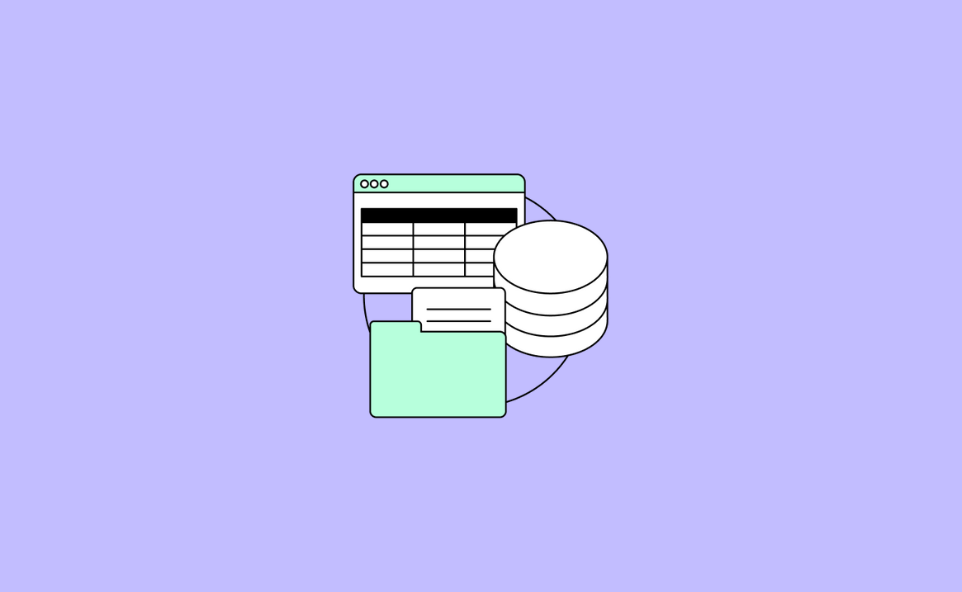
In an unforgivingly competitive business environment, many organisations have only two choices – innovate faster than ever before or face imminent disruption.
As a result, many are turning to AI and machine learning (ML) to unlock the potential of their data.
But there is often one major roadblock.
The data itself.
Large amounts of data are essential for driving ML models and training large language models (LLMs).
While many organisations already have this data, the underlying problem is they can’t safely use it.
Strict data privacy regulations and security concerns can prohibit the use of sensitive customer data like PII, financial information, or healthcare records for model training.
Using real customer data to train or fine-tune models poses serious risks, including the potential for data leaks and models memorising information.
But models built on synthetic or open-source datasets produce generic results, as they frequently fail to capture the true nature of the real data.
This can cause major difficulties when such a model is released to production.
Using poor-quality training data can result in:
▪ Low model accuracy and high error rates
▪ An ongoing need for costly human intervention and tuning
▪ An increased risk of non-compliance or security breaches
At the same time, the demand for reliable data is surging as AI adoption continues to skyrocket. A report by Grand View Research predicts that the AI market will grow at an annual rate of 37.3% between 2023 and 2030.1
This presents a major hurdle for some organisations racing to adopt ML. Without access to relevant data, ML initiatives end up stalled or delivering lack lustre results.
Breaking the data bottleneck with DataMasque
With DataMasque, you can use your own real-world data to build accurate machine learning models, while ensuring privacy and compliance.
Our proprietary data masking technology rapidly de-identifies sensitive columns in databases and files by replacing identifiable information with anonymised but realistic values. This provides privacy protection while retaining the essential patterns and relationships in the data critical for effective model training.
DataMasque has several key capabilities:
▪ Automated scanning to detect and classify sensitive information.
▪ Configurable data masking rules tailored to specific privacy risks.
▪ Referential integrity preserved by masking data consistently across all databases and environments.
▪ Ability to mask streaming data via APIs to enable real-time ML model training.
▪ Contro lover synthetic data accuracy, variability, and masking to meet specific training needs.
DataMasque can provide a privacy-safe replica of real-world data in the volume and variety required for robust MLtraining, overcoming the typical limitations of small sample sizes or unrealistic dummy data.
Our approach is superior to manual masking techniques or trying to source relevant open datasets which are costly, inaccurate, and insecure.
DataMasque has many use cases
▪ Banks, insurers, and capital markets firms have huge troves of customer financial data and transactional records that are incredibly valuable for training ML models.
However, using this highly sensitive PII and account data for ML is prohibited outright or heavily restricted under regulations like GDPR, CCPA, and HIPAA.
DataMasque can de-identify this data, enabling financial institutions to tap into some of their richest data assets to drive AI innovation.
With DataMasque, firms like retail banks and insurance companies can safely leverage years of customer account history for producing models that detect fraud, predict client needs, and optimise marketing strategies.
▪ Telecommunications providers also have vast subscriber data that is tightly regulated.
Using DataMasque telecommunications companies can draw on usage data such as call and messaging logs or network events, to power next-best action recommendations and improve customer experience through AI assistants.
▪ Healthcare organisations hold an ever-increasing amount of sensitive patient data.
DataMasque can unlock electronic health records and genomic data, including FHIR records, to accelerate ML use cases while still safeguarding patient confidentiality.
Wherever GDPR, CCPA, or HIPAA regulation sapply, DataMasque’s privacy-preserving ML data preparation capability enables you to fully capitalise on all of AI’s capabilities.
Are you ready to discover the potential of your data?